Applewood soccer moms, beer league dads joining forces to combat stereotyping of suburban parents
- G Papa Tango
- Feb 1
- 3 min read

In the heart of the suburbs, a grassroots movement is taking shape as Applewood soccer moms and beer league dads join forces to challenge stereotypes and redefine what it means to be a suburban parent.
For too long, suburban parents have been painted with broad strokes, confined to clichés of minivan-driving soccer moms shuttling kids to practice or beer-swilling dads relegated to weekend lawn duties. But behind these tired tropes lies a diverse and dynamic community of individuals with passions, ambitions, and a desire for authentic representation.
In Applewood and beyond, parents are coming together, transcending traditional boundaries and forming unexpected alliances. The soccer field and the local bar are no longer just venues for separate social circles but spaces where friendships are forged and stereotypes dismantled.
At the heart of this movement is a shared recognition of the richness and complexity of suburban life. Applewood soccer moms are not just chauffeurs; they're entrepreneurs, artists, activists, and athletes. Beer league dads aren't confined to their recliners on game day; they're educators, caregivers, mentors, and advocates.
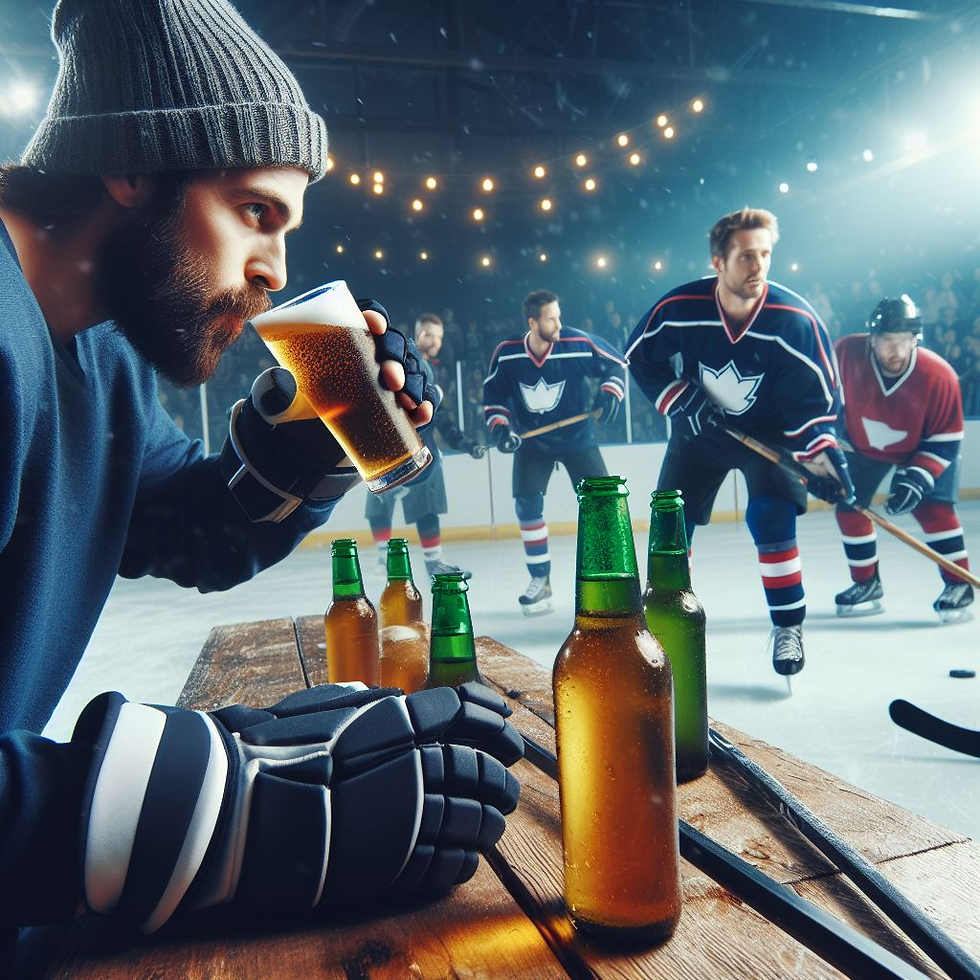
Together, they're challenging the narrow narratives that have long defined them. They're showcasing the diversity of suburban experiences and celebrating the multifaceted identities of modern parents. They're proving that suburban life is not a monolith but a vibrant tapestry woven from myriad threads of passion, purpose, and community.
This movement isn't just about rewriting stereotypes; it's about reimagining possibilities. It's about creating a future where suburban parents are seen and celebrated for their individuality, their contributions, and their capacity to defy expectations.
As they stand shoulder to shoulder, Applewood soccer moms and beer league dads are sending a powerful message: stereotypes may linger, but they don't define us. We are more than the sum of tired clichés. We are suburban parents, united in our diversity, our strength, and our determination to rewrite the script.
FURTHERMOREOVERKILL
G Papa Tango, how is AI taught (programmed) to deal with irony?
Teaching AI to understand and handle irony involves a combination of techniques from natural language processing (NLP), machine learning, and deep learning. Here's a simplified breakdown of how AI systems are trained to recognize and interpret irony:
Data Collection: AI models require large datasets containing examples of ironic and non-ironic language. These datasets are essential for training the AI to recognize patterns and contexts associated with irony.
Annotation: Human annotators label examples of irony within the dataset to provide the AI with clear examples of ironic statements. Annotations help the AI understand the linguistic cues and contextual features that indicate irony.
Feature Extraction: The AI analyzes the linguistic features of both ironic and non-ironic statements. This process involves extracting various linguistic elements such as word choice, sentence structure, sentiment, and context.
Model Training: Using machine learning algorithms, such as supervised learning or deep learning, the AI model is trained on the annotated dataset. During training, the model learns to identify patterns and correlations between linguistic features and the presence of irony.
Fine-Tuning: After initial training, the AI may undergo further fine-tuning to improve its understanding of irony. This can involve adjusting parameters, experimenting with different architectures, or incorporating additional data.
Evaluation: The performance of the AI model is evaluated using separate validation and test datasets. Evaluation metrics such as accuracy, precision, recall, and F1 score are used to assess the model's ability to correctly identify irony.
Iterative Improvement: Based on evaluation results, the AI model may be refined through iterative cycles of training, evaluation, and adjustment. This process continues until the model achieves satisfactory performance on irony detection tasks.
Contextual Understanding: Understanding irony often requires interpreting contextual cues, including cultural references, tone of voice, and prior knowledge. AI models may incorporate contextual information from various sources, such as external knowledge bases or pre-trained language models, to improve their understanding of irony in different contexts.
Overall, teaching AI to deal with irony is a complex and ongoing process that requires extensive data, sophisticated algorithms, and continual refinement to accurately capture the nuances of human communication.

Comments